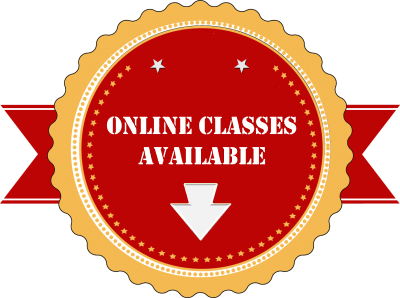
Both online and in-person classes are available for local and non-local students, based on their convenience.
-“Our service is global.”
For details, Call: 9886692401/9845166723
DHS Informatics providing latest 2024-2025 IEEE projects on AI, ML & DL for the final year engineering students. DHS Informatics trains all students to develop their project with good idea what they need to submit in college to get good marks. DHS Informatics offers placement training in Bangalore and the program name is OJT – On Job Training, job seekers as well as final year college students can join in this placement training program and job opportunities in their dream IT companies. We are providing IEEE projects for BE / B.TECH, M.TECH, MCA, BCA, DIPLOMA students from more than two decades.
-
Python Final year CSE projects in Bangalore
Artificial Intelligence (AI)
Artificial Intelligence (AI) is like a brain for machines. It helps them think and make decisions, kind of like how we humans do. But instead of using flesh and blood, AI uses computer programs. These programs learn from data they’re given, getting smarter over time. AI is all around us, from the voice assistants in our phones to the recommendation systems on streaming platforms. It helps make our lives easier by automating tasks, like sorting emails or driving cars. AI can also help us solve complex problems, like predicting the weather or diagnosing diseases. However, there are also concerns about AI, such as privacy issues and job automation. Overall, AI is a powerful tool with the potential to revolutionize many aspects of our lives.
Machine Learning (ML)
Machine Learning (ML) is a type of technology that helps computers learn from data and improve their performance over time without being explicitly programmed. Think of it like teaching a child to recognize objects, but instead of showing pictures and explaining, you give the computer lots of data, and it figures out the patterns by itself.
For example, if you want a computer to recognize pictures of cats, you would show it thousands of images labeled as “cat” or “not cat.” The computer analyzes these images, learns the differences, and creates a model. Once trained, it can look at a new picture and tell you whether it’s a cat or not.
There are different types of ML:
1. Supervised Learning: The computer is given labeled data (like the cat example) and learns to predict labels for new data.
2. Unsupervised Learning: The computer finds patterns in data without labels. For instance, it might group customers with similar buying habits together.
3. Reinforcement Learning: The computer learns by making decisions and receiving rewards or penalties. It’s like training a dog with treats.
ML is used in many everyday applications, such as email spam filters, recommendation systems on streaming services, voice assistants like Siri or Alexa, and even self-driving cars. It’s a powerful tool that helps improve technology by making it smarter and more efficient.
Deep Learning (DL)
Deep Learning (DL) is a type of machine learning, which is a branch of artificial intelligence. It focuses on teaching computers to learn from data and make decisions, similar to how humans do.
Imagine you want to teach a computer to recognize pictures of cats. In the past, you would have to manually program the computer to look for specific features like whiskers, ears, and tails. With deep learning, you don’t have to do this. Instead, you feed the computer lots of pictures of cats and not-cats. The computer then uses this information to learn by itself what makes a cat a cat.
Deep learning uses structures called neural networks, which are designed to mimic the human brain. These networks have layers of interconnected nodes (neurons) that process the information. When you provide an input (like a picture), the data passes through these layers, and the network learns to recognize patterns and make predictions.
This technology is behind many things we use today, such as voice assistants like Siri or Alexa, recommendation systems on Netflix or YouTube, and even self-driving cars. Deep learning helps these systems improve over time as they are exposed to more data, making them smarter and more accurate.
Abstract : Information hiding aims to embed secret data into the multimedia, such as image, audio, video, and text. In this paper, two new quantum information hiding approaches are put forward. A quantum stenography approach is proposed to hide a quantum secret image into a quantum cover image. The quantum secret image is encrypted first using a controlled-NOT gate to demonstrate the security of the embedded data. The encrypted secret image is embedded into the quantum cover image using the two most and least significant qubits. In addition, a quantum image watermarking approach is presented to hide a quantum watermark gray image into a quantum carrier image. The quantum watermark image, which is scrambled by utilizing Arnold’s cat map, is then embedded into the quantum carrier image using the two least and most significant qubits. Only the watermarked image and the key are sufficient to extract the embedded quantum watermark image. The proposed novelty has been illustrated using a scenario of sharing medical imagery between two remote hospitals. The simulation and analysis demonstrate that the two newly proposed approaches have excellent visual quality and high embedding capacity y and security.
Abstract : Reversible data hiding in encrypted images (RDHEI) is an effective technique to embed data in the encrypted domain. An original image is encrypted with a secret key and during or after its transmission, it is possible to embed additional information in the encrypted image, without knowing the encryption key or the original content of the image. During the decoding process, the secret message can be extracted and the original image can be reconstructed. In the last few years, RDHEI has started to draw research interest. Indeed, with the development of cloud computing, data privacy has become a real issue. However, none of the existing methods allows us to hide a large amount of information in a reversible manner. In this paper, we propose a new reversible method based on MSB (most significant bit) prediction with a very high capacity. We present two approaches, these are: high capacity reversible data hiding approach with correction of prediction errors (CPE-HCRDH) and high capacity reversible data hiding approach with embedded prediction errors (EPE-HCRDH). With this method, regardless of the approach used, our results are better than those obtained with current state of the art methods, both in terms of reconstructed image quality and embedding capacity
Abstract : The aim of this paper is to maximize the range of the access control of visual secret sharing (VSS) schemes encrypting multiple images. First, the formulation of access structures for a single secret is generalized to that for multiple secrets. This generalization is maximal in the sense that the generalized for-mulation makes no restrictions on access structures; in particular, it includes the existing ones as special cases. Next, a sufficient condition to be satisfied by the encryption of VSS schemes realizing an access structure for multiple secrets of the most general form is introduced, and two constructions of VSS schemes with encryption satisfying this condition are provided. Each of the two constructions has its advantage against the other; one is more general and can generate VSS schemes with strictly better contrast and pixel expansion than the other, while the other has a straightforward implementation. Moreover, for threshold access structures, the pixel expansions of VSS schemes generated by the latter construction are estimated and turn out to be the same as those of the existing schemes called the threshold multiple-secret visual cryptographic schemes. Finally, the optimality of the former construction is examined, giving that there exist access structures for which it generates no optimal VSS schemes.
Abstract : One of the becoming popular bio metric modalities is the palm print. This bio metric modality is rich with information, such as minutiae, ridges, wrinkles, and creases. This research team is interested to investigate the creases for bio metric identification. The palm print images in this research have been captured by using a commercially available consumer scanner. For each palm print image, two square regions on the palm print image are extracted for bio metric identification purpose. One of the regions is from the hyperthyroid region, while the another is from the inter digital region. Due to misalignment of the hand, the process of extraction of these regions is tedious and time-consuming. Therefore, in this paper, a computer-aided method has been proposed to simplify the extraction process. The user only needs to mark two points on the palm print image. Based on these points, the palm print image will be aligned, and those two regions are extracted automatically.
Abstract: This paper presents fast categorization or classification of images on an animal data set using different classification algorithm in combination with manifold learning algorithms. The paper will focus on comparing the effects of different non-linear dimensional reduction algorithms on speed and accuracy of different classification algorithms. It examines how manifold learning algorithms can improve classification speed by reducing the number of features in the vector representation of images while keeping the classification accuracy high.
DHS Informatics believes in students’ stratification, we first brief the students about the technologies and type of Image Processing projects and other domain projects. After complete concept explanation of the IEEE Image Processing projects, students are allowed to choose more than one IEEE Image Processing projects for functionality details. Even students can pick one project topic from Image Processing and another two from other domains like Image Processing, data mining, image process, information forensic, big data, Image Processing, Image Processing, data science, block chain etc. DHS Informatics is a pioneer institute in Bangalore / Bengaluru; we are supporting project works for other institute all over India. We are the leading final year project centre in Bangalore / Bengaluru and having office in five different main locations Jayanagar, Yelahanka, Vijayanagar, RT Nagar & Indiranagar.
We allow the ECE, CSE, ISE final year students to use the lab and assist them in project development work; even we encourage students to get their own idea to develop their final year projects for their college submission.
DHS Informatics first train students on project related topics then students are entering into practical sessions. We have well equipped lab set-up, experienced faculties those who are working in our client projects and friendly student coordinator to assist the students in their college project works.
We appreciated by students for our Latest IEEE projects & concepts on final year Image Processing projects for ECE, CSE, and ISE departments.
Latest IEEE 2024-2025 projects on Image Processing with real time concepts which are implemented using Java, MATLAB, and NS2 with innovative ideas. Final year students of computer Image Processing, computer science, information science, electronics and communication can contact our corporate office located at Jayanagar, Bangalore for Image Processing project details.
IMAGE PROCESSING
Image processing is processing of images using mathematical operations by using any form of signal processing for which the input is an image, a series of images, such as a photograph, the output of image processing may be either an image or a set of characteristics or parameters related to the image.
Most image-processing techniques involve isolating the individual color planes of an image and treating them as two-dimensional signal and applying standard signal-processing techniques to them. Images are also processed as three-dimensional signals with the third dimension being time or the z-axis.
Image processing usually refers to digital image processing, but optical and analog image processing also are possible. This article is about general techniques that apply to all of them. The acquisition of images (producing the input image in the first place) is referred to as imaging.
Java Final year CSE projects in Bangalore
- Java Information Forensic / Block Chain B.E Projects
- Java Cloud Computing B.E Projects
- Java Big Data with Hadoop B.E Projects
- Java Networking & Network Security B.E Projects
- Java Data Mining / Web Mining / Cyber Security B.E Projects
- Java DataScience / Machine Learning B.E Projects
- Java Artificaial Inteligence B.E Projects
- Java Wireless Sensor Network B.E Projects
- Java Distributed & Parallel Networking B.E Projects
- Java Mobile Computing B.E Projects
Android Final year CSE projects in Bangalore
- Android GPS, GSM, Bluetooth & GPRS B.E Projects
- Android Embedded System Application Projetcs for B.E
- Android Database Applications Projects for B.E Students
- Android Cloud Computing Projects for Final Year B.E Students
- Android Surveillance Applications B.E Projects
- Android Medical Applications Projects for B.E
Embedded Final year CSE projects in Bangalore
- Embedded Robotics Projects for M.tech Final Year Students
- Embedded IEEE Internet of Things Projects for B.E Students
- Embedded Raspberry PI Projects for B.E Final Year Students
- Embedded Automotive Projects for Final Year B.E Students
- Embedded Biomedical Projects for B.E Final Year Students
- Embedded Biometric Projects for B.E Final Year Students
- Embedded Security Projects for B.E Final Year
MatLab Final year CSE projects in Bangalore
- Matlab Image Processing Projects for B.E Students
- MatLab Wireless Communication B.E Projects
- MatLab Communication Systems B.E Projects
- MatLab Power Electronics Projects for B.E Students
- MatLab Signal Processing Projects for B.E
- MatLab Geo Science & Remote Sensors B.E Projects
- MatLab Biomedical Projects for B.E Students