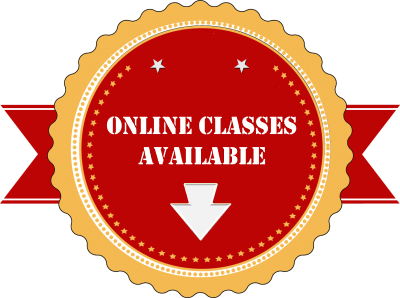
For Outstation Students, we are having online project classes both technical and coding using net-meeting software
For details, Call: 9886692401/9845166723
DHS Informatics providing latest 2021-2022 IEEE projects on Data science for the final year engineering students. DHS Informatics trains all students to develop their project with good idea what they need to submit in college to get good marks. DHS Informatics offers placement training in Bangalore and the program name is OJT – On Job Training, job seekers as well as final year college students can join in this placement training program and job opportunities in their dream IT companies. We are providing IEEE projects for B.E / B.TECH, M.TECH, MCA, BCA, DIPLOMA students from more than two decades.
Python Final year CSE projects in Bangalore
DATA SCIENCE PROJECTS
Abstract: Fraudulent behavior in drinking water consumption is a significant problem facing water supplying companies and agencies. This behavior results in a massive loss of income and forms the highest percentage of non-technical loss. Finding efficient measurements for detecting fraudulent activities has been an active research area in recent years. Intelligent data mining techniques can help water supplying companies to detect these fraudulent activities to reduce such losses. This research explores the use of two classification techniques (SVM and KNN) to detect suspicious fraud water customers. The main motivation of this research is to assist Yarmouk Water Company (YWC) in Irbid city of Jordan to overcome its profit loss. The SVM based approach uses customer load profile attributes to expose abnormal behavior that is known to be correlated with non-technical loss activities. The data has been collected from the historical data of the company billing system. The accuracy of the generated model hit a rate of over 74% which is better than the current manual prediction procedures taken by the YWC. To deploy the model, a decision tool has been built using the generated model. The system will help the company to predict suspicious water customers to be inspected on site.
Abstract: As a typical latent factor model, Matrix Factorization (MF) has demonstrated its great effectiveness in recommender systems. Users and items are represented in a shared low-dimensional space so that the user preference can be modeled by linearly combining the item factor vector V using the user-specific coefficients U. From a generative model perspective, U and V are drawn from two independent Gaussian distributions, which is not so faithful to the reality. Items are produced to maximally meet users’ requirements, which makes U and V strongly correlated. Meanwhile, the linear combination between U and V forces a bisection (one-to-one mapping), which thereby neglects the mutual correlation between the latent factors. In this paper, we address the upper drawbacks, and propose a new model, named Correlated Matrix Factorization (CMF). Technically, we apply Canonical Correlation Analysis (CCA) to map U and V into a new semantic space. Besides achieving the optimal fitting on the rating matrix, one component in each vector (U or V) is also tightly correlated with every single component in the other. We derive efficient inference and learning algorithms based on variational EM methods. The effectiveness of our proposed model is comprehensively verified on four public data sets. Experimental results show that our approach achieves competitive performance on both prediction accuracy and efficiency compared with the current state of the art.
Abstract: Due to the flexibility in modelling data heterogeneity, heterogeneous information network (HIN) has been adopted to characterize complex and heterogeneous auxiliary data in recommended systems, called HIN based recommendation. It is challenging to develop effective methods for HIN based recommendation in both extraction and exploitation of the information from HINs. Most of HIN based recommendation methods rely on path based similarity, which cannot fully mine latent structure features of users and items. In this paper, we propose a novel heterogeneous network embedding based approach for HIN based recommendation, called HERec. To embed HINs, we design a meta-path based random walk strategy to generate meaningful node sequences for network embedding. The learned node embeddings are first transformed by a set of fusion functions, and subsequently integrated into an extended matrix factorization (MF) model. The extended MF model together with fusion functions are jointly optimized for the rating prediction task. Extensive experiments on three real-world datasets demonstrate the effectiveness of the HERec model. Moreover, we show the capability of the HERec model for the cold-start problem, and reveal that the transformed embedding information from HINs can improve the recommendation performance.
Abstract: Nowadays, a big part of people rely on available content in social media in their decisions (e.g., reviews and feedback on a topic or product). The possibility that anybody can leave a review provides a golden opportunity for spammers to write spam reviews about products and services for different interests. Identifying these spammers and the spam content is a hot topic of research, and although a considerable number of studies have been done recently toward this end, but so far the methodologies put forth still barely detect spam reviews, and none of them show the importance of each extracted feature type. In this paper, we propose a novel framework, named NetSpam, which utilizes spam features for modeling review data sets as heterogeneous information networks to map spam detection procedure into a classification problem in such networks. Using the importance of spam features helps us to obtain better results in terms of different metrics experimented on real-world review data sets from Yelp and Amazon Web sites. The results show that NetSpam outperforms the existing methods and among four categories of features, including review-behavioral, user-behavioral, review-linguistic, and user-linguistic, the first type of features performs better than the other categories.
Abstract:Nowadays, heart disease is a common and frequently present disease in the human body and it’s also hunted lots of humans from this world. Especially in the USA, every year mass people are affected by this disease after that in India also. Doctor and clinical research said that heart disease is not a suddenly happen disease it’s the cause of continuing irregular lifestyle and different body’s activity for a long period after then it’s appeared in sudden with symptoms. After appearing those symptoms people seek for a treat in hospital for taken different test and therapy but these are a little expensive. So awareness before getting appeared in this disease people can get an idea about the patient condition from this research result. This research collected data from different sources and split that data into two parts like 80% for the training dataset and the rest 20% for the test dataset. Using different classifier algorithms tried to get better accuracy and then summarize that accuracy. These algorithms are namely Random Forest Classifier, Decision Tree Classifier, Support Vector Machine, k-nearest neighbor, Logistic Regression, and Naive Bayes. SVM, Logistic Regression, and KNN gave the same and better accuracy as other algorithms. This paper proposes a development that which factor is vulnerable to heart disease given basic prefix like sex, glucose, Blood pressure, Heart rate, etc. The future direction of this paper is using different devices and clinical trials for the real-life experiment.
Abstract:This study was conducted to apply supervised machine learning methods in opinion mining online customer reviews. First, the study automatically collected 39,976 traveler reviews on hotels in Vietnam on Agoda.com website, then conducted the training with machine learning models to find out which model is most compatible with the training dataset and apply this model to forecast opinions for the collected dataset. The results showed that Logistic Regression (LR), Support Vector Machines (SVM) and Neural Network (NN) methods have the best performance in opinion mining in Vietnamese language. This study is valuable as a reference for applications of opinion mining in the field of business.
Abstract:The area of medical science has attracted great attention from researchers. Several causes for human early mortality have been identified by a decent number of investigators. The related literature has confirmed that diseases are caused by different reasons and one such cause is heart-based sicknesses. Many researchers proposed idiosyncratic methods to preserve human life and help health care experts to recognize, prevent and manage heart disease. Some of the convenient methodologies facilitate the expert’s decision but every successful scheme has its own restrictions. The proposed approach robustly analyze an act of Hidden Markov Model (HMM), Artificial Neural Network (ANN), Support Vector Machine (SVM), and Decision Tree J48 along with the two different feature selection methods such as Correlation Based Feature Selection (CFS) and Gain Ratio. The Gain Ratio accompanies the Ranker method over a different group of statistics. After analyzing the procedure the intended method smartly builds Naive Bayes processing that utilizes the operation of two most appropriate processes with suitable layered design. Initially, the intention is to select the most appropriate method and analyzing the act of available schemes executed with different features for examining the statistics.
Abstract:In the modern era, many reasons for agricultural plant disease due to unfavorable weather conditions. Many reasons that influence disease in agricultural plants include variety/hybrid genetics, the lifetime of plants at the time of infection, environment(soil, climate), weather (temperature, wind, rain, hail, etc), single versus mixed infections, and genetics of the pathogen populations. Due to these factors, diagnosis of plant diseases at the early stages can be a difficult task. Machine Learning (ML) classification techniques such as Naïve Bayes (NB) and Neural Network (NN) techniques were compared to develop a novel technique to improve the level of accuracy
Abstract:Brain is the controlling center of our body. With the advent of time, newer and newer brain diseases are being discovered. Thus, because of the variability of brain diseases, existing diagnosis or detection systems are becoming challenging and are still an open problem for research. Detection of brain diseases at an early stage can make a huge difference in attempting to cure them. In recent years, the use of artificial intelligence (AI) is surging through all spheres of science, and no doubt, it is revolutionizing the field of neurology. Application of AI in medical science has made brain disease prediction and detection more accurate and precise. In this study, we present a review on recent machine learning and deep learning approaches in detecting four brain diseases such as Alzheimer’s disease (AD), brain tumor, epilepsy, and Parkinson’s disease. 147 recent articles on four brain diseases are reviewed considering diverse machine learning and deep learning approaches, modalities, datasets etc. Twenty-two datasets are discussed which are used most frequently in the reviewed articles as a primary source of brain disease data. Moreover, a brief overview of different feature extraction techniques that are used in diagnosing brain diseases is provided. Finally, key findings from the reviewed articles are summarized and a number of major issues related to machine learning/deep learning-based brain disease diagnostic approaches are discussed. Through this study, we aim at finding the most accurate technique for detecting different brain diseases which can be employed for future betterment.
Abstract:Chronic Kidney Disease is one of the most critical illness nowadays and proper diagnosis is required as soon as possible. Machine learning technique has become reliable for medical treatment. With the help of a machine learning classifier algorithms, the doctor can detect the disease on time. For this perspective, Chronic Kidney Disease prediction has been discussed in this article. Chronic Kidney Disease dataset has been taken from the UCI repository. Seven classifier algorithms have been applied in this research such as artificial neural network, C5.0, Chi-square Automatic interaction detector, logistic regression, linear support vector machine with penalty L1 & with penalty L2 and random tree. The important feature selection technique was also applied to the dataset. For each classifier, the results have been computed based on (i) full features, (ii) correlation-based feature selection, (iii) Wrapper method feature selection, (iv) Least absolute shrinkage and selection operator regression, (v) synthetic minority over-sampling technique with least absolute shrinkage and selection operator regression selected features, (vi) synthetic minority over-sampling technique with full features. From the results, it is marked that LSVM with penalty L2 is giving the highest accuracy of 98.86% in synthetic minority over-sampling technique with full features. Along with accuracy, precision, recall, F-measure, area under the curve and GINI coefficient have been computed and compared results of various algorithms have been shown in the graph. Least absolute shrinkage and selection operator regression selected features with synthetic minority over-sampling technique gave the best after synthetic minority over-sampling technique with full features. In the synthetic minority over-sampling technique with least absolute shrinkage and selection operator selected features, again linear support vector machine gave the highest accuracy of 98.46%. Along with machine learning models one deep neural network has been applied on the same dataset and it has been noted that deep neural network achieved the highest accuracy of 99.6%
Abstract:In Bangladesh potato is one of the major crops. Potato cultivation has been very popular in Bangladesh for the last few decades. But potato production is being hampered due to some diseases which are increasing the cost of farmers in potato production. However, some potato diseases are hampering potato production that is increasing the cost of farmers. Which is disrupting the life of the farmer. An automated and rapid disease detection process to increase potato production and digitize the system. Our main goal is to diagnose potato disease using leaf pictures that we are going to do through advanced machine learning technology. This paper offers a picture that is processing and machine learning based automated systems potato leaf diseases will be identified and classified. Image processing is the best solution for detecting and analyzing these diseases. In this analysis, picture division is done more than 2034 pictures of unhealthy potato and potato’s leaf, which is taken from openly accessible plant town information base and a few pre-prepared models are utilized for acknowledgment and characterization of sick and sound leaves. Among them, the program predicts with an accuracy of 99.23% in testing with 25% test data and 75% train data. Our output has shown that machine learning exceeds all existing tasks in potato disease detection.
Abstract:With the technological advancement in the field of digital transformation, the use of the internet and social media has increased immensely. Many people use these platforms to share their views, opinions and experiences. Analyzing such information is significant for any organization as it apprises the organization to understand the need of their customers. Sentiment analysis is an intelligible way to interpret the emotions from the textual information and it helps to determine whether that emotion is positive or negative. This paper outlines the data cleaning and data preparation process for sentiment analysis and presents experimental findings that demonstrates the comparative performance analysis of various classification algorithms. In this context, we have analyzed various machine learning techniques (Support Vector Machine, and Multinomial Naive Bayes) and deep learning techniques (Bidirectional Encoder Representations from Transformers, and Long Short-Term Memory) for sentiment analysis
Abstract:Email is the most used source of official communication method for business purposes. The usage of the email continuously increases despite of other methods of communications. Automated management of emails is important in the today’s context as the volume of emails grows day by day. Out of the total emails, more than 55 percent is identified as spam. This shows that these spams consume email user time and resources generating no useful output. The spammers use developed and creative methods in order to fulfil their criminal activities using spam emails, Therefore, it is vital to understand different spam email classification techniques and their mechanism. This paper mainly focuses on the spam classification approached using machine learning algorithms. Furthermore, this study provides a comprehensive analysis and review of research done on different machine learning techniques and email features used in different Machine Learning approaches. Also provides future research directions and the challenges in the spam classification field that can be useful for future researchers.
Abstract:Heart disease causes a significant mortality rate around the world, and it has become a health threat for many people. Early prediction of heart disease may save many lives; detecting cardiovascular diseases like heart attacks, coronary artery diseases etc., is a critical challenge by the regular clinical data analysis. Machine learning (ML) can bring an effective solution for decision making and accurate predictions. The medical industry is showing enormous development in using machine learning techniques. In the proposed work, a novel machine learning approach is proposed to predict heart disease. The proposed study used the Cleveland heart disease dataset, and data mining techniques such as regression and classification are used. Machine learning techniques Random Forest and Decision Tree are applied. The novel technique of the machine learning model is designed. In implementation, 3 machine learning algorithms are used, they are 1. Random Forest, 2. Decision Tree and 3. Hybrid model (Hybrid of random forest and decision tree). Experimental results show an accuracy level of 88.7% through the heart disease prediction model with the hybrid model. The interface is designed to get the user’s input parameter to predict the heart disease, for which we used a hybrid model of Decision Tree and Random Forest
Abstract:Heart disease is one of the major cause of mortality in the world today. Prediction of cardiovascular disease is a critical challenge in the field of clinical data analysis. With the advanced development in machine learning (ML), artificial intelligence (AI) and data science has been shown to be effective in assisting in decision making and predictions from the large quantity of data produced by the healthcare industry. ML approaches has brought lot of improvements and broadens the study in medical field which recognizes patterns in the human body by using various algorithms and correlation techniques. One such reality is coronary heart disease, various studies gives impression into predicting heart disease with ML techniques. Initially ML was used to find degree of heart failure, but also used to identify significant features that affects the heart disease by using correlation techniques. There are many features/factors that lead to heart disease like age, blood pressure, sodium creatinine, ejection fraction etc. In this paper we propose a method to finding important features by applying machine learning techniques. The work is to design and develop prediction of heart disease by feature ranking machine learning. Hence ML has huge impact in saving lives and helping the doctors, widening the scope of research in actionable insights, drive complex decisions and to create innovative products for businesses to achieve key goals.
Abstract:Today’s pandemic situation has transformed the way of educating a student. Education is undertaken remotely through online platforms. In addition to the way the online course contents and online teaching, it has also changed the way of assessments. In online education, monitoring the attendance of the students is very important as the presence of students is part of a good assessment for teaching and learning. Educational institutions have adopting online examination portals for the assessments of the students. These portals make use of face recognition techniques to monitor the activities of the students and identify the malpractice done by them. This is done by capturing the students’ activities through a web camera and analyzing their gestures and postures. Image processing algorithms are widely used in the literature to perform face recognition. Despite the progress made to improve the performance of face detection systems, there are issues such as variations in human facial appearance like varying lighting condition, noise in face images, scale, pose etc., that blocks the progress to reach human level accuracy. The aim of this study is to increase the accuracy of the existing face recognition systems by making use of SVM and Eigenface algorithms. In this project, an approach similar to Eigenface is used for extracting facial features through facial vectors and the datasets are trained using Support Vector Machine (SVM) algorithm to perform face classification and detection. This ensures that the face recognition can be faster and be used for online exam monitoring.
Abstract:Brain is the controlling center of our body. With the advent of time, newer and newer brain diseases are being discovered. Thus, because of the variability of brain diseases, existing diagnosis or detection systems are becoming challenging and are still an open problem for research. Detection of brain diseases at an early stage can make a huge difference in attempting to cure them. In recent years, the use of artificial intelligence (AI) is surging through all spheres of science, and no doubt, it is revolutionizing the field of neurology. Application of AI in medical science has made brain disease prediction and detection more accurate and precise. In this study, we present a review on recent machine learning and deep learning approaches in detecting four brain diseases such as Alzheimer’s disease (AD), brain tumor, epilepsy, and Parkinson’s disease. 147 recent articles on four brain diseases are reviewed considering diverse machine learning and deep learning approaches, modalities, datasets etc. Twenty-two datasets are discussed which are used most frequently in the reviewed articles as a primary source of brain disease data. Moreover, a brief overview of different feature extraction techniques that are used in diagnosing brain diseases is provided. Finally, key findings from the reviewed articles are summarized and a number of major issues related to machine learning/deep learning-based brain disease diagnostic approaches are discussed. Through this study, we aim at finding the most accurate technique for detecting different brain diseases which can be employed for future betterment.
IEEE DATA SCIENCE PROJECTS (2020-2021)
DHS Informatics believes in students’ stratification, we first brief the students about the technologies and type of Data Science projects and other domain projects. After complete concept explanation of the IEEE Data Science projects, students are allowed to choose more than one IEEE Data Science projects for functionality details. Even students can pick one project topic from Data Science and another two from other domains like Data Science,Data mining, image process, information forensic, big data, Data Mining, block chain etc. DHS Informatics is a pioneer institute in Bangalore / Bengaluru; we are supporting project works for other institute all over India. We are the leading final year project centre in Bangalore / Bengaluru and having office in five different main locations Jayanagar, Yelahanka, Vijayanagar, RT Nagar & Indiranagar.
We allow the ECE, CSE, ISE final year students to use the lab and assist them in project development work; even we encourage students to get their own idea to develop their final year projects for their college submission.
DHS Informatics first train students on project related topics then students are entering into practical sessions. We have well equipped lab set-up, experienced faculties those who are working in our client projects and friendly student coordinator to assist the students in their college project works.
We appreciated by students for our Latest IEEE projects & concepts on final year Data Mining projects for ECE, CSE, and ISE departments.
Latest IEEE 2021-2022 projects on Data Mining with real time concepts which are implemented using Java, MATLAB, and NS2 with innovative ideas. Final year students of computer Data Mining, computer science, information science, electronics and communication can contact our corporate office located at Jayanagar, Bangalore for Data Science project details.
DATA SCIENCE
Data Science is mining knowledge from data, Involving methods at the intersection of machine learning, statistics, and database systems. Its the powerful new technology with great potential to help companies focus on the most important information in their data warehouses. We have the best in class infrastructure, lab set up , Training facilities, And experienced research and development team for both educational and corporate sectors.
Data Science is the process of searching huge amount of data from different aspects and summarize it to useful information. Data Science is logical than physical subset. Our concerns usually implicate mining and text based classification on Data Science projects for Students.
The usages of variety of tools associated to data analysis for identifying relationships in data are the process for Data Science. Our concern support data mining projects for IT and CSE students to carry out their academic research projects.
Data Science is the process of searching huge amount of data from different aspects and summarize it to useful information. Data Science is logical than physical subset. Our concerns usually implicate mining and text based classification on data Science projects for Students. The usages of variety of tools associated to data analysis for identifying relationships in data are the process for data Science. Our concern support data Science projects for IT and CSE students to carry out their academic research projects.
Relational Statics
The popularity of the term “data science” has exploded in business environments and academia, as indicated by a jump in job openings. However, many critical academics and journalists see no distinction between data science and statistics. Writing in Forbes, Gil Press argues that data science is a buzzword without a clear definition and has simply replaced “business analytics” in contexts such as graduate degree programs.In the question-and-answer section of his keynote address at the Joint Statistical Meetings of American Statistical Association, noted applied statistician Nate Silver said, “I think data-scientist is a sexed up term for a statistician….Statistics is a branch of science. Data scientist is slightly redundant in some way and people shouldn’t berate the term statistician.”Similarly, in business sector, multiple researchers and analysts state that data scientists alone are far from being sufficient in granting companies a real competitive advantage and consider data scientists as only one of the four greater job families companies require to leverage big data effectively, namely: data analysts, data scientists, big data developers and big data engineers.
On the other hand, responses to criticism are as numerous. In a 2014 Wall Street Journal article, Irving Wladawsky-Berger compares the data science enthusiasm with the dawn of computer science. He argues data science, like any other interdisciplinary field, employs methodologies and practices from across the academia and industry, but then it will morph them into a new discipline. He brings to attention the sharp criticisms computer science, now a well respected academic discipline, had to once face.Likewise, NYU Stern’s Vasant Dhar, as do many other academic proponents of data science,argues more specifically in December 2013 that data science is different from the existing practice of data analysis across all disciplines, which focuses only on explaining data sets. Data science seeks actionable and consistent pattern for predictive uses.This practical engineering goal takes data science beyond traditional analytics. Now the data in those disciplines and applied fields that lacked solid theories, like health science and social science, could be sought and utilized to generate powerful predictive models.
Java Final year CSE projects in Bangalore
- Java Information Forensic / Block Chain B.E Projects
- Java Cloud Computing B.E Projects
- Java Big Data with Hadoop B.E Projects
- Java Networking & Network Security B.E Projects
- Java Data Mining / Web Mining / Cyber Security B.E Projects
- Java DataScience / Machine Learning B.E Projects
- Java Artificaial Inteligence B.E Projects
- Java Wireless Sensor Network B.E Projects
- Java Distributed & Parallel Networking B.E Projects
- Java Mobile Computing B.E Projects
Android Final year CSE projects in Bangalore
- Android GPS, GSM, Bluetooth & GPRS B.E Projects
- Android Embedded System Application Projetcs for B.E
- Android Database Applications Projects for B.E Students
- Android Cloud Computing Projects for Final Year B.E Students
- Android Surveillance Applications B.E Projects
- Android Medical Applications Projects for B.E
Embedded Final year CSE projects in Bangalore
- Embedded Robotics Projects for M.tech Final Year Students
- Embedded IEEE Internet of Things Projects for B.E Students
- Embedded Raspberry PI Projects for B.E Final Year Students
- Embedded Automotive Projects for Final Year B.E Students
- Embedded Biomedical Projects for B.E Final Year Students
- Embedded Biometric Projects for B.E Final Year Students
- Embedded Security Projects for B.E Final Year
MatLab Final year CSE projects in Bangalore
- Matlab Image Processing Projects for B.E Students
- MatLab Wireless Communication B.E Projects
- MatLab Communication Systems B.E Projects
- MatLab Power Electronics Projects for B.E Students
- MatLab Signal Processing Projects for B.E
- MatLab Geo Science & Remote Sensors B.E Projects
- MatLab Biomedical Projects for B.E Students